Detecting Generative AI Content
On deepfakes, authenticity, and the President’s Executive Order on AI
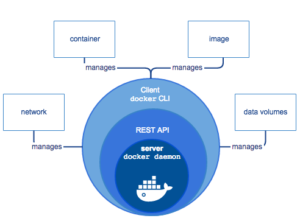
One of the many interesting ethical issues that comes with the advances of generative AI is detection of the product of models. It’s a practical issue as well, for those of us who consume media. Is this thing I am reading or looking at the product of a person’s thoughtful work, or just words or images probabilistically generated to appeal to me? Does it matter? If so, what do we do?
The Meaning of Indistinguishable Content
When we talk about content being difficult or impossible to detect as AI generated, we’re getting into something akin to a Turing Test. Say that I give you a paragraph of text or an image file. If I ask you, “was this produced by a human being or a machine learning model?”, and you can’t accurately tell, then we’ve reached the point where we need to think about these issues.
In many areas we’re close to this, especially with GPT-4, but even with less sophisticated models, depending on what kind of prompt we use and the volume of context. If we have a long document from a GPT model, it’s likely going to be easier to detect that it’s not human generated, because every new word is a chance for the model to do something that a regular person wouldn’t do. Same for a video or high-res image — the more chances for pixellation or the uncanny valley to show up, the more opportunity for us to spot the fake.
It also seems clear to me that as we get more familiar with model generated content, we’re going to get better at identifying tell tale signs of AI involvement in the content. Just like I described several weeks ago in my explanation of how Generative Adversarial Networks work, we are in something of a GAN relationship with generative AI ourselves. The models are working on creating the most human-like content possible, and we are increasing our ability to tell that it’s not human. It’s like a race where each side is working to outwit the other.